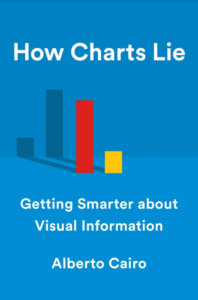
Continuing in my pandemic reading of data books, next up is “How Charts Lie: Getting Smarter about Visual Information” by Alberto Cairo. (I didn’t plan to be a predominately book review blog, but I need a way to channel the pandemic anxiety, so here we are.)
This book is a little different than other visualization books I’ve been reading because it focuses on visual literacy (which Cairo calls “graphicacy”) instead of chart design. Because charts appear by their nature more authoritative (they show “facts” and make such information easy to understand), we need to train ourselves to critically assess the information displayed. This book provides the framework for an individual to engage with and dissect the charts we regularly see in the news and on social media and decide what’s accurate.
Cairo uses his experience as a chart designer and chart consumer to break down the major ways that charts lie. Each type of lie gets covered in its own chapter in the book:
- Poor design
- Displaying dubious data
- Displaying insufficient data
- Concealing or confusing uncertainty
- Suggesting misleading patterns
You’ll notice that these mistakes aren’t all about chart design; many chart issues concern the data that’s being visualized, including everything from displaying percentages instead of absolute numbers on a map to vetting data sources. Cairo provides ways to think through the many mistakes that are made in data selection, because even the prettiest and easiest-to-read chart can lie to us by getting the data wrong .
What’s nice about the book is that it doesn’t assume that charts are intentionally lying to us. Sometimes designers make honest mistakes and sometimes trade-offs have to be made. Cairo walks the reader through exemplar visualizations and shows us how different choices affect the accuracy and design of the chart. By discussing the data selection and visualization decision process as well as showing how these choices affect the final design, Cairo provides the reader with the mental scaffolding to critically assess charts.
As with any data book, Cairo uses plenty of examples throughout this book. What I found interesting is how many of these examples were drawn from recent politics; the book actually starts by dissecting a graphic that Donald Trump shared in April 2017. While I appreciate the American cultural touchstones (and it’s nice to rage at some of the bad charts we’ve seen in recent years), I do worry that this book will lose some of its relevance over time.
Overall, this is a good book for any information consumer to read and will also help visualization designers learn to avoid pitfalls and assess design trade-offs. I would also recommend it to my fellow librarians who do information literacy instruction; the visual literacy discussed in this book is a perfect compliment to the work we’re already doing with students around assessing text-based resources.